AI cannot predict the future. But companies keep trying (and failing).
A new paper on how AI companies make false promises and how we can challenge them
While the world gushes over large language models and text-to-image tools, a far more consequential kind of AI tool has been proliferating: AI for making decisions about individuals based on predictions about their future behavior. We call this predictive optimization.
Governments, banks, and employers use predictive optimization to make life-changing decisions such as whom to investigate for child maltreatment, whom to approve for a loan, and whom to hire. Companies sell predictive optimization with the promise of more accurate, fair, and efficient decisions. They claim it does away with human discretion entirely. And since predictive optimization relies entirely on existing data, it is cheap: no additional data is needed.
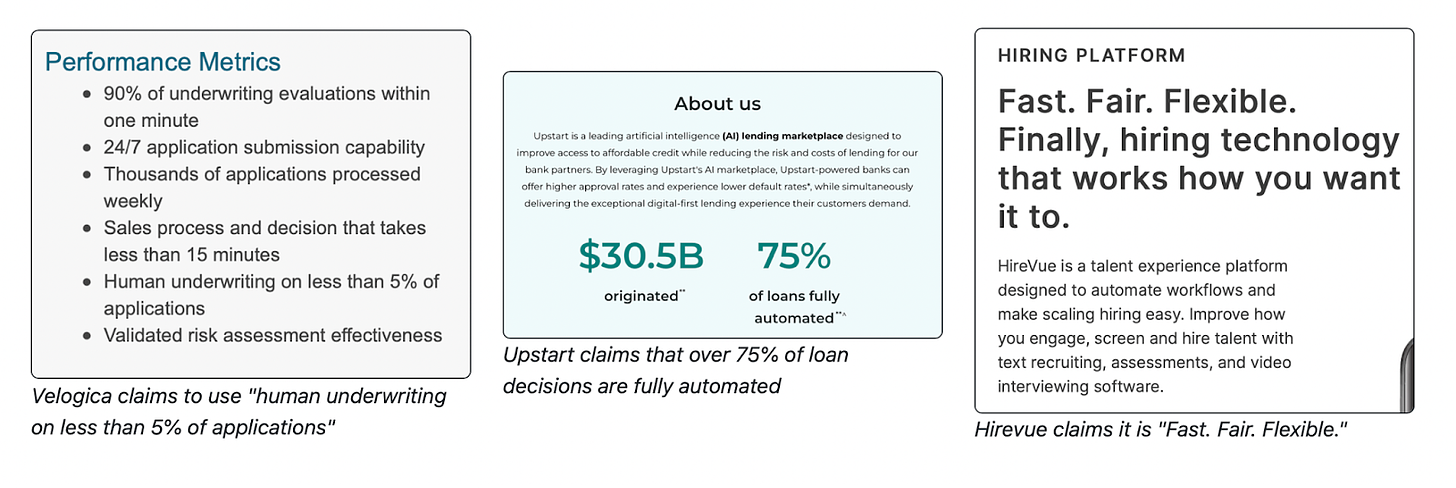
But do these claims hold up? Our hunch was: no. But hunches are merely the beginning of a research project. Over the last year, together with Angelina Wang and Solon Barocas, we investigated predictive optimization in-depth:Â
We read 387 news articles, Kaggle competition descriptions, and reports to find 47 real-world applications of predictive optimization. From these 47, we chose the eight most consequential applications.Â
We then read over 100 papers on the shortcomings of AI in making decisions about people and selected seven critiques that challenged developers' claims of accuracy, fairness, and efficiency.Â
Finally, we checked if these seven critiques apply to our chosen applications by reviewing past literature and giving our own arguments where necessary.
The table below presents our main results. Each row in the table is one of the eight applications we chose; each column is a critique. Our main empirical finding is that each critique applies to every application we selected.
Our analysis hints that these shortcomings apply uniformly across applications of predictive optimization, even beyond the eight that we looked at in detail. The entire category of AI tools that make decisions by predicting an individual's future is likely similarly flawed and warrants caution.
What can we do to push back against predictive optimization? Our main suggestion: shift the burden of evidence onto developers of predictive optimization. Given that these applications overwhelmingly fail to work as promised, the bar for releasing a new application must be much higher.Â
To aid in this task, we provide a rubric of 27 questions based on our seven critiques. Advocates and civil rights activists can use it to challenge the deployment of predictive optimization. Developers can use it to check if their application suffers from common pitfalls and take steps to remedy them. And the next time you come across predictive optimization in the wild, you can use the rubric to evaluate if the claims developers make are possible in the first place.
We're happy that regulators are starting to pay attention to whether developers are keeping their promises. Holding developers accountable requires political will and organized effort from many stakeholders, including academics, activists, and regulators.
The paper is titled Against Predictive Optimization: On the Legitimacy of Decision-Making Algorithms that Optimize Predictive Accuracy. Read the draft paper here and a summary of our findings here.Â
Further reading
Inioluwa Deborah Raji et al. argue that policy debates are derailed by the assumption that AI tools work as intended. The authors taxonomize known failures of AI and argue that policy discussions on AI should begin with the question: does it even work?
Amanda Coston et al. ask: when is a predictive algorithm justifiable? They highlight that questions about the validity of predictive algorithms are overlooked and outline how they can be incorporated into a deliberative process for determining whether to use predictive algorithms.
Great content, very useful, thanks!
The closest I have seen an "AI" (or modestly sized neural net) "predict" was in a guitar-to-MIDI translator box. At the lowest fundamental frequencies, waiting for a whole (let alone several, for improved accuracy) waveform periods to be captured takes too long to be perceived as instantaneous (the MIDI communication and synthesizer played will add further delay), and the signal of a freshly attacked string doesn't look that clean either. But NN was able to reasonably reliably detect the vibration frequency with a fraction of a period captured - which some might want to call predicting the future (continuation of not very sinusoidal waveform whose shapes vary wildly with how the string is attacked and what it did before). One might say that's just pattern recognition, but I guess there is overlap between that and "predicting the future".