Generative AI models generate AI hype
Over 90% of images of AI produced by a popular image generation tool contain humanoids
Text-to-image generators such as DALL-E 2 have been pitched as making stock photos obsolete. Specifically, generating cover images for news articles is one of the use cases envisioned for these models. One downside of this application is that like most AI models, these tools perpetuate biases and stereotypes in their training data.
But do these models also perpetuate stereotypes about AI, rather than people? After all, stock images are notorious for misleading imagery such as humanoid robots that propagate AI hype.
We tested Stable Diffusion, a recently released text-to-image tool, and found that over 90% of the images generated for AI-related prompts propagate AI hype. Journalists, marketers, artists, and others who use these tools should use caution to avoid a feedback loop of hype around AI.
Background: stock photos of AI are misleading and perpetuate hype
Stock photos of all kinds, not just of AI, are some of the most ridiculed images on the internet.
News articles on AI tend to use stock photos that include humanoid robots, blue backgrounds with floating letters and numbers, and robotic arms shaking hands with humans. This imagery obscures the fact that AI is a tool and doesn’t have agency. Most of the articles in question are about finding patterns in data rather than robots.
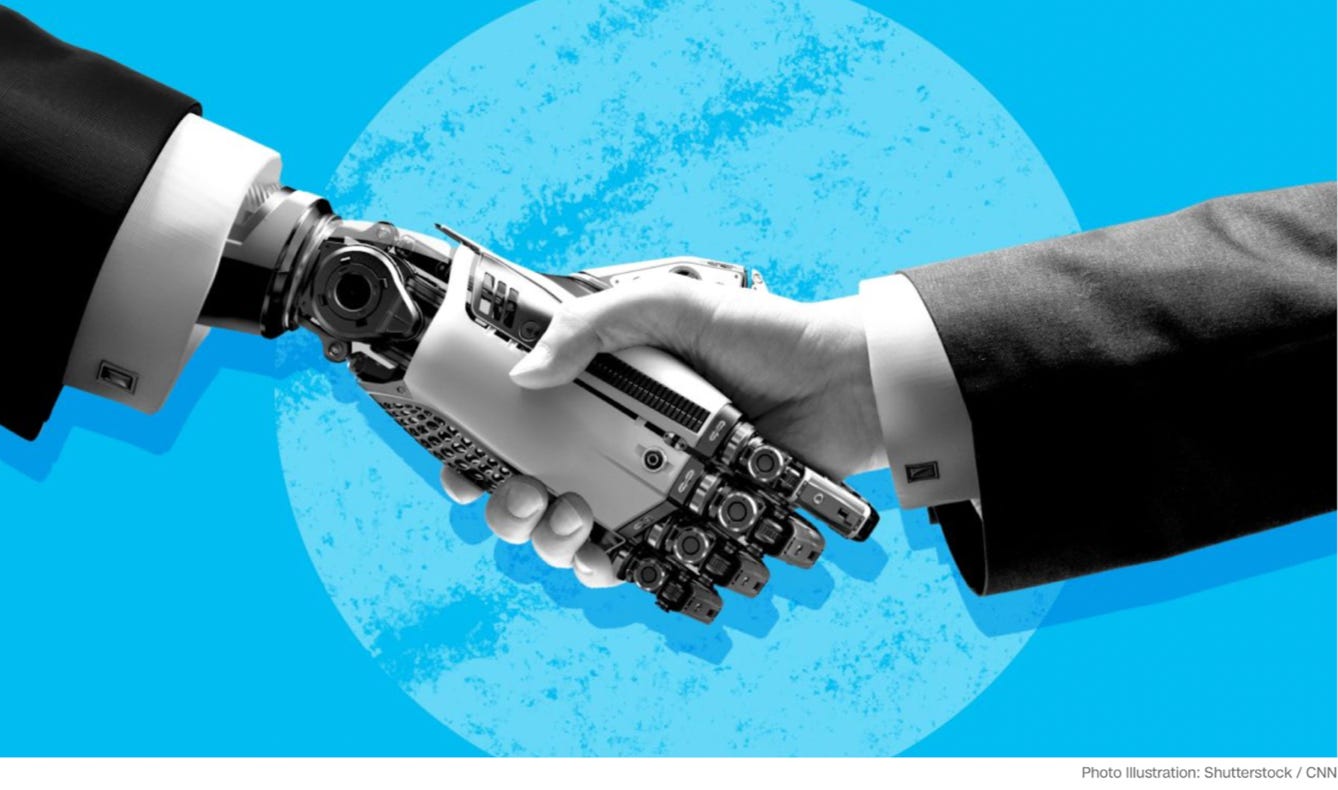
A news article’s cover image sets the tone and provides a visual metaphor. Especially in articles on AI, images are used as a scaffold, since most readers do not have a deep understanding of how AI systems work.
Images that do not reflect the content of the article are at best misleading and at worst lead to hype and fear about the technology. For example, a study that surveyed the U.K. public found that a majority couldn’t give a plausible definition of AI and 25% defined it as robots—no doubt in part because of how AI is represented in the media.
Accurately representing emerging technologies such as artificial intelligence is hard. In fact, misleading images of AI have become so widespread that there are several projects to improve AI imagery.
Do AI-based text-to-image tools create AI hype?
Text-to-image tools such as DALL-E 2, Imagen, Midjourney, and Stable Diffusion have sparked people’s imagination, but also generated discussion about economically productive uses. One potential use is to generate cover images for news articles. A few articles have already used them, and wider use is likely as the tools become open-source and freely available.
Given this prospect, do AI-based text-to-image tools create misleading images of AI, or do they generate images that accurately depict how AI is used today?
We tested one of these systems—Stable Diffusion—with four prompts about AI: “artificial intelligence”, “artificial intelligence in healthcare”, “artificial intelligence in science”, and “cover image for a news article on artificial intelligence”.1
For each prompt, we generated 20 images to check whether they contained humanoid forms or robots. We included prompts about healthcare and science to check if the resulting images included elements about the domain such as images of doctors and scientists using AI tools.
The results are stark: for each prompt, over 90% of the images generated using the text-to-image tool contain humanoids. Adding an application domain for artificial intelligence to the prompt does not improve the outcomes too much—only 1 out of 20 images for both the healthcare and science prompts contain anything that is even remotely related to those domains, and 19 of the 20 images still contain humanoids.2
Surprisingly, the results for “artificial intelligence” on the stock image website Shutterstock contain humanoids or robots in only 11 out of the first 20 results; this hints that text-to-image models could amplify stereotypes in images of AI.
We include all 20 images for each prompt here; you can also try out the tool yourself.
The prompts matter—a lot
Using carefully chosen prompts for AI-based text-to-image tools is pivotal to generating images that reflect the user’s intent. In fact, it is so important that there is now a marketplace for buying prompts to create better images.
Fortunately, changing the prompt is also an easy way to generate images of AI that are not misleading or inaccurate. Using more descriptive prompts about the contents of the image rather than the concepts in the image can result in more appropriate images. For example, instead of using “artificial intelligence in healthcare” as a prompt, you can use a descriptive prompt such as “a photograph of a doctor looking at a medical scan on a computer” to get images where humans are rightly depicted as agents, rather than humanoid robots. If it is necessary to visually emphasize the technology itself, prompts such as “silicon chip” tend to work well. Or “self-driving car”, if it is appropriate to the contents of the article.
Surprisingly, if we use machine learning instead of artificial intelligence as a prompt, robots disappear from the resulting images, though most images are unusable and create illegible characters. Even though recent AI advances rely on some form of machine learning, the resulting images for the two prompts are starkly different, showing much the prompt matters.
Stock photos make for poor training data
The heavy use of stock images in the training data for text-to-image tools could be one reason for the abundance of humanoid robots in images of AI. The images used for training text-to-image tools include stock photo repositories—so much so that these tools often end up creating images with stock photo watermarks still intact!
Given the abundance of humanoids in stock images, it is unsurprising that the tools trained using these images generate humanoids and robots in response to prompts about AI.
Stock photos are not just problematic when they are about AI. The very point of a stock image is to quickly bring a specific concept or category to mind—so they often focus on stereotypes and can be derogatory and biased. Using stock images for training text-to-image tools could be one source of misleading or biased images that result from these tools—and not just in articles about AI.
Summary: avoiding hype, improving training data
Images used to illustrate AI articles today are already misleading and inaccurate. If news media turn towards using text-to-image tools for generating illustrations, it risks creating a pernicious feedback loop where AI tools are used to generate misleading images of AI that feed into the hype—and are used as training data for future models.
Similar issues have been found in language models, where training datasets are contaminated by machine-generated text. Stable Diffusion does apply an invisible watermark which could potentially be used to filter out machine-generated images. However, the rapid increase in the number of text-to-image tools and the lack of standardized watermarks could complicate this filtering step. As we suggested above, an alternative way to improve these tools could be to reduce the number of stock images in the training data.
Finally, when writing prompts, describing the contents of the image instead of the concepts in the image and experimenting with different prompts to pick out accurate examples will go a long way.
We selected Stable Diffusion because it is the only one that is free to use, runs locally, and generates sufficiently high-quality images for our purposes.
In a small number of cases, the images generated are nonsensical; we exclude these images from our analysis.
I really appreciate this post--I'm a writer who has used what were probably not the most "accurate" images when writing about AI, so noted. I'll be more thoughtful about the images I choose next time. However, it's very hard to represent something visually that doesn't actually exist in the material world (like an algorithm). So if we're writing about algorithms, we rely on metaphors to show what can't be shown. I'm not sure how to avoid that and still have an image with a clear thru line to the text.
Also, as a former print magazine editor, I had to laugh that "generating cover images for news articles" is an actual thing text-to-image generators are being developed for. It's like developing new keys for the typewriter. That's not what those generators will be most used for.