Something weird happened on November 19, 2019.
When a professor shares scholarly slides online, they are usually intended for a niche group of peers. You’d be lucky if 20 people looked at them. But that day, the slides Arvind released went viral. They were downloaded tens of thousands of times and his tweets about them were viewed 2 million times.

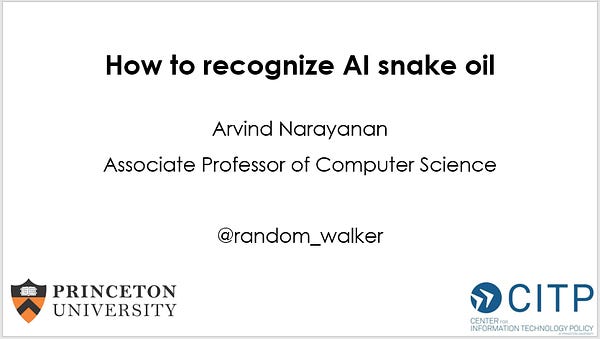
Once the shock wore off, it was clear why the topic had touched a nerve. Most of us suspect that a lot of AI around us is fake, but don’t have the vocabulary or the authority to question it. After all, it’s being peddled by supposed geniuses and trillion-dollar companies. But a computer science professor calling it out gave legitimacy to those doubts. That turned out to be the impetus that people needed to share their own skepticism.
Within two days, Arvind’s inbox had 40-50 invitations to turn the talk into an article or even a book. But he didn’t think he understood the topic well enough to write a book. He didn’t want to do it unless he had a book’s worth of things to say, and he didn’t want to simply trade on the popularity of the talk.
That’s where Sayash comes in. The two of us have been working together for the last two years to understand the topic better.
In the Fall of 2020, Sayash took a course on Limits to Prediction, offered by Arvind and sociology professor Matt Salganik. It asked critically: given enough data, is everything predictable?
It was a course at the cutting edge of research, and the instructors learned together with the students. Through our readings and critical analysis, we confirmed our hunch that in virtually every attempt to predict some aspect of the future of the social world, forecasters have run into strong limits. That’s just as true for predicting a child’s outcomes in school as massive geopolitical events. It didn’t matter much which methods they used. Besides, the same few limitations kept recurring. That’s very strong evidence that there are inherent limits.
The only exception to this pattern came from political scientists who were using AI to predict civil wars. According to a series of recent papers, AI far outperformed older statistical methods at this task.
We were curious, and decided to find out why. What we found instead was that each paper claiming the superior performance of AI methods suffered from errors. When the errors were corrected, they performed no better than 20 year old methods. This shocked us: peer-reviewed studies which had been cited hundreds of times had built consensus around an invalid claim.
These findings confirmed Sayash's previous experiences at Facebook, where he saw how easy it was to make errors when building predictive models and be over-optimistic about their efficacy. Errors could arise due to many subtle reasons and often weren’t caught until the model was deployed.
After three years of research, separately and together, we’re ready to share what we’ve learned. Hence this book. But the book isn’t just about sharing knowledge. AI is being used to make impactful decisions about us every day, so broken AI can and does wreck lives and careers. Of course, not all AI is snake oil — far from it — so the ability to distinguish genuine progress from hype is critical for all of us. Perhaps our book can help.
We hear the clock ticking as we write this. The dubious uses of AI that we were concerned about, such as in the areas of criminal risk prediction and hiring, have massively expanded in the last few years. New ones are introduced every week. The list of harms keeps multiplying. Mark Zuckerberg promised Congress that AI would solve the problem of keeping harmful content off the platform. The role of social media in the January 6 attack reminds us how poorly those efforts are currently working.
Meanwhile the public discourse about AI has gone beyond parody, getting distracted by debates such as whether AI is sentient. Every day developers of AI tools make grad claims about its efficacy without any public (let alone peer reviewed) evidence. And, as our research has shown, we need to be skeptical of even the peer reviewed evidence in this area.
Fortunately, there’s a big community pushing back against AI’s harms. In the last five years, the idea that AI is often biased and discriminatory has gone from a fringe notion to one that is widely understood. It has gripped advocates, policy makers, and (reluctantly) companies. But addressing bias isn’t nearly enough. It shouldn’t distract us from the more fundamental question of whether and when AI works at all. We hope to elevate that question in the public consciousness.
This isn’t a regular book. We have already written a lot about this topic and plan to share our ideas with you every step of the way. We’re excited that Princeton University Press has agreed to publish our book. We were taken aback when our editor said this topic deserves a trade book with wide circulation. We only have experience with academic publishing, and have never done something like this before. But we’re excited to be doing it and we hope you follow along.
If you’re concerned about AI snake oil, there are many things you can do today. Take a look at the overview of our book. Educate your friends and colleagues about the issue. Read the AI news skeptically — in fact, we’ll soon be sharing our analysis of how pervasive AI hype is in the media.
When you’re making purchase decisions about AI-based products and services, be sure to ask critical questions, and don’t be suckered into buying snake oil. If they can’t give you a coherent explanation you can understand, the problem is not you, it’s them. It might be because the tech doesn’t work.
As a citizen, exercise your right to protest when you’re subject to algorithmic decisions without transparency. Engage with the democratic process to resist surveillance AI.
And finally, a plea to our fellow techies and engineers: refuse to build AI snake oil.
Looking forward to this - pre-ordered the book! Loved the MLST pod appearance
This is such a great initiative. I feel the same way at work, things are expected to happen magically using "AI". Now I have something to share when I hear this next time.
Your work is going to train a lot of smart minds and give them whole new perspective or even careers. Love it and looking forward to more of it!! Thanks again for doing this.